The strongest antibiotic HALICIN ever discovered by humans for the first time fully exploited by AI
Antibiotics have been the cornerstone of modern medicine since the advent of penicillin. However, over time, bacteria develop resistance to antibiotics, which requires drug researchers to continuously develop new antibiotics.
But the reality is that private pharmaceutical companies have had little success in discovering new antibiotics due to a lack of economic incentives, making the antibiotic problem worse. Studies have predicted that if no immediate action is taken to develop new antibiotics, the number of deaths from drug-resistant infections will reach 10 million a year by 2050.
Over the past few decades, researchers have used a variety of traditional methods to unearth new antibiotics, but many times, they have found the same molecules over and over again, so discovery of new antibiotics has been a struggle. In this context, the biomedical community urgently needs new methods to help discover new antibiotics.
Addressing the most dangerous pathogen on the WHO 'wanted list'
To address these issues, researchers from the MIT Center for Synthetic Biology developed a deep learning method that can predict the activity of antibiotic molecules, identifying a powerful new antibiotic molecule called halicin from more than 107 million molecules. Halicin works against a variety of bacteria, such as tuberculosis, as well as strains that are considered untreatable. Moreover, the newly discovered molecule is structurally very different from known antibiotic molecules.
In mouse experiments, the molecule showed antibacterial activity against a variety of pathogens, including Clostridioides difficile, Enterobacteriaceae, and Acinetobacter baumannii, which is "extensively drug resistant" and in urgent need of new antibiotics (Acinetobacter baumannii). Among them, the latter two are listed as high-priority pathogens by the World Health Organization (WHO) and are two of the three major research targets for new antibiotics.
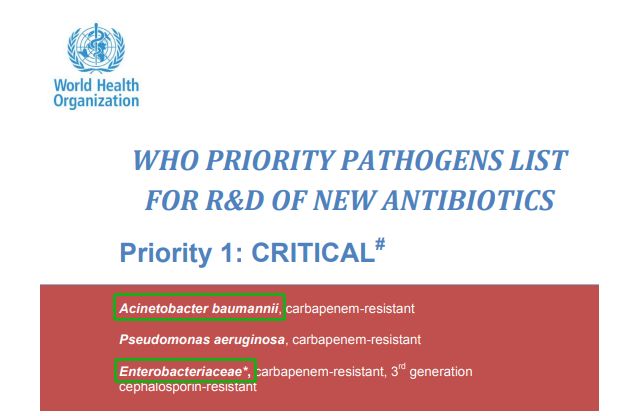
While there have been previous examples of using artificial intelligence for some antibiotic discoveries, the research team emphasizes that this latest discovery is based on the identification of a completely new class of antibiotics from scratch without any prior assumptions.
At present, this research work is led by Jim Collins, a synthetic biologist at the Massachusetts Institute of Technology (MIT), and has been published in the authoritative academic journal "Cell" and has become the cover article of the current issue.
"In terms of antibiotic discovery, this is definitely a first," said Regina Barzilay, an MIT machine learning expert and one of the authors of the study.
"We want to be able to develop a platform that will allow humans to harness the power of artificial intelligence to usher in a new era of antibiotic drug discovery. I think halicin is one of the most powerful antibiotics ever discovered by humans," added MIT team bioengineer James Collins road. "It showed remarkable activity against a large number of drug-resistant pathogens."
Paper link: https://www.cell.com/cell/fulltext/S0092-8674(20)30102-1
Jacob Durrant, a computational biologist at the University of Pittsburgh in Pennsylvania, said the study was significant because the team not only identified candidate molecules, but also validated potential molecules in animal experiments. More importantly, this method has a certain generalizability and can be applied to other types of drug research, such as the treatment of cancer or neurodegenerative diseases.
discovery process
In their search for new antibiotics, the researchers trained a deep neural network to find molecules that inhibit the growth of E. coli. During the training, they used 2,335 molecules known to have antibacterial activity, including about 300 approved antibiotics and 800 natural products derived from plants, animals and microorganisms.
In this study, the model did not need to know assumptions about the drug's mechanism, nor did it require the annotation of chemical groups to perform predictions. The model is able to learn new patterns unknown to human experts.
After training, the researchers used the model to perform molecular screening in a library called the "Drug Repurposing Hub," which contains about 6,000 drug molecules that are in the research phase to fight human diseases.
The purpose of the screen was to see which molecules worked against E. coli, and to pick out those that didn't look the same as regular antibiotics. From these results, another 100 candidate molecules were selected for physical experiments,
As a result, they found that a molecule called "halicin", which is in the research phase for diabetes treatment, works well. In experiments in mice, the molecule showed antibacterial activity against a variety of pathogens, including Clostridium difficile, Enterobacteriaceae, and Acinetobacter baumannii, which are "extensively resistant" and in urgent need of new antibiotics.
Pictorial abstract of the paper
Graph modeling of antibiotic molecules
Overall, the role of the neural network is to predict the ultimate inhibitory ability of different molecular structures on Escherichia coli. In normal thinking, we need a dataset where the input features are the components and structures of molecules, annotated as the ability to inhibit E. coli. The advantage of a neural network is that it can represent a molecule with a vector it learns itself, rather than a hand-designed feature vector.
In this paper, the researchers show that directed information transfer networks can predict the properties of molecules directly from their graph structure, where atoms can be represented as nodes and chemical bonds can be represented as edges. For each chemical molecule, the researchers build a molecular map for each component's SMILES expression, where SMILES is a specification that unambiguously describes the molecular structure in ASCII strings.
When the eigenvector is initialized, it is further divided into atomic eigenvectors and chemical bond eigenvectors. The leftmost neural network is modeled below. The top is a molecular graph. The third atom and the fourth atom have a chemical bond (Bond) to the second atom. The two black vectors below represent these two connect. And so on, the red vector represents the bond where the 2nd atom is connected to the 1st atom.
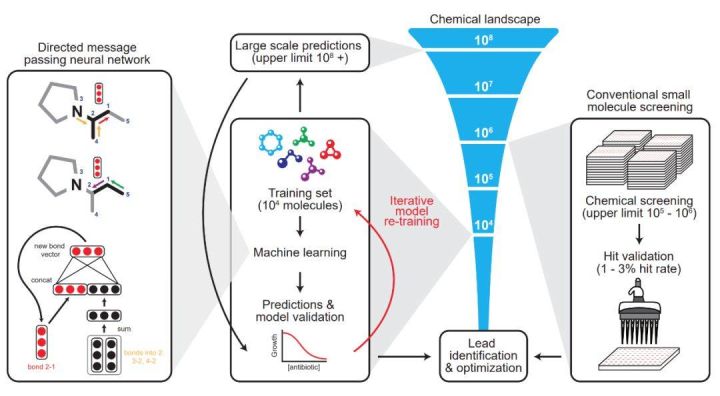
The model applies a series of information transfer steps that aggregate information from adjacent atoms and chemical bonds to understand local molecular chemistry. During each information transfer process of the directed information transfer network, the chemical bond eigenvectors can be updated by summing the adjacent chemical bond eigenvectors and feeding it into a nonlinear single-layer neural network.
After a fixed number of information transfer steps, the various feature vectors learned throughout the molecule are summed into a single vector and fed to a feed-forward neural network to predict the inhibitory effect of the chemical molecule on E. coli. The latter FFN is a very conventional two-classification problem, so the most important part of the entire antibiotic molecular modeling is the graphical model of the first step: how to use graphs and vectors to represent complex relationships between molecules.
This groundbreaking work marks a paradigm shift in the approach to antibiotic discovery and, more generally, new drug discovery. In the future, the MIT researchers also plan to use deep learning models to design new antibiotics and optimize existing molecules.
In fact, the discovery of antibiotic molecules is only the tip of the iceberg of the application of machine learning in the field of drug mining. Scholars all over the world are trying to use machine learning technology to speed up the development of new drugs. Taking the new drug research and development of the new coronavirus as an example, some AI drug research and development companies have begun to try to use a variety of deep learning models to mine drug molecules (for example, Inke Intelligence uses more than 20 models to find drugs that inhibit the key proteases of the new coronavirus. Molecules), and related results are also being announced one after another.
Thanks to the great discoveries of great medical scientists, discover more antibacterial drugs and disinfecting products for the well-being and destiny of mankind.